Perception of national campaigns during crisis
Prof. Mao Kezhi is studying the impact and potential long-term consequences of the #SGUnited national campaign, which could provide insights to better shape future resilience policies and campaigns.
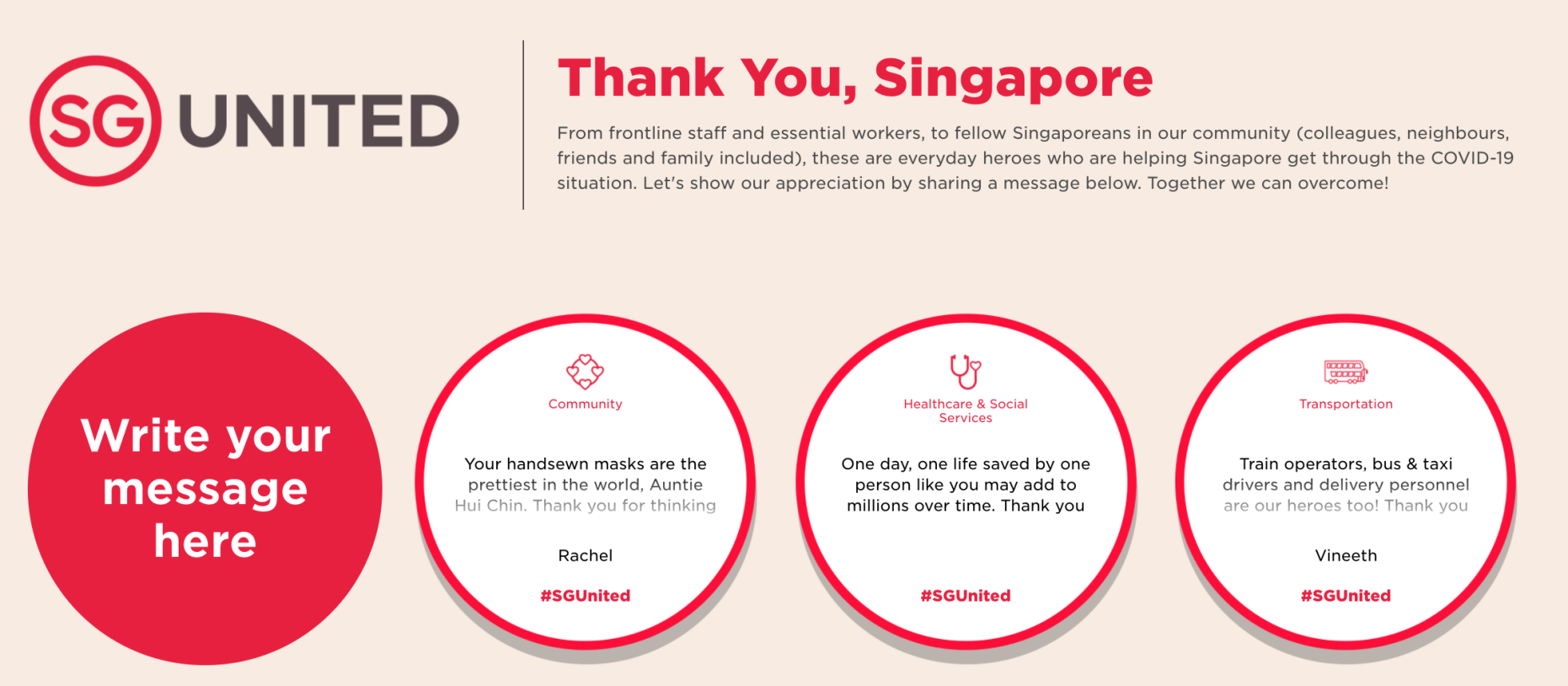
In the face of crises, messages of unity and reinforcing a common identity are common recommendations to build resilience. National campaigns can help to rally people together, which could go a long way in building community resilience. In Singapore, the #SGUnited campaign was rolled out during the COVID-19 pandemic. The question is: What are Singaporeans’ perceptions towards the campaign?
To understand Singaporeans’ perceptions, external page Prof. Mao Kezhi and his team at NTU are examining public sentiments of this campaign, in collaboration with the Home Team Behavioural Sciences Centre (HTBSC) under the Ministry of Home Affairs. Prof. Mao leads the Distributed Cognition for Social Resilience research cluster and the Automated Hazard Detection for Social Resilience research module in the Future Resilient Systems (FRS) programme.
The team conducts sentiment analysis by studying statements in social media posts (Facebook and Twitter), local news websites, and forums to identify emotions and opinions to identify positive, neutral, or negative sentiments.Sentiment analysis is a subfield of natural language processing (NLP) that aims to identify and classify opinions within text. It has been a topic of great interest in recent years because of the availability of a large volume of texts expressing opinions in review sites, forums, newspapers, and social media.
The three approaches to sentiment analysis include rule-based approach, statistical machine learning approach, and neural network and deep learning approach. In this project, the team adopts the neural network approach, in particular the convolutional neural network in combination with neural language models, as they often achieve the best performance and require minimal human intervention. Specifically, the team is employing neural language models and transfer learning in this study.
In general, huge amounts of resources are required to train deep neural network models, which makes transfer learning a popular method in deep learning. In transfer learning, a model developed for one task is reused as the starting point for a model on another task. Therefore, in spite of the lack of labelled training data for specific sentiment analysis, labelled training data for other sentiment analysis tasks like Amazon customer review dataset, movie review dataset IMBD etc., could be used as starting points.
The findings of this three-month project could provide useful insights on the impact and potential long-term consequences of such campaigns and help the relevant agencies in shaping future resilience policies and campaigns post-COVID-19.