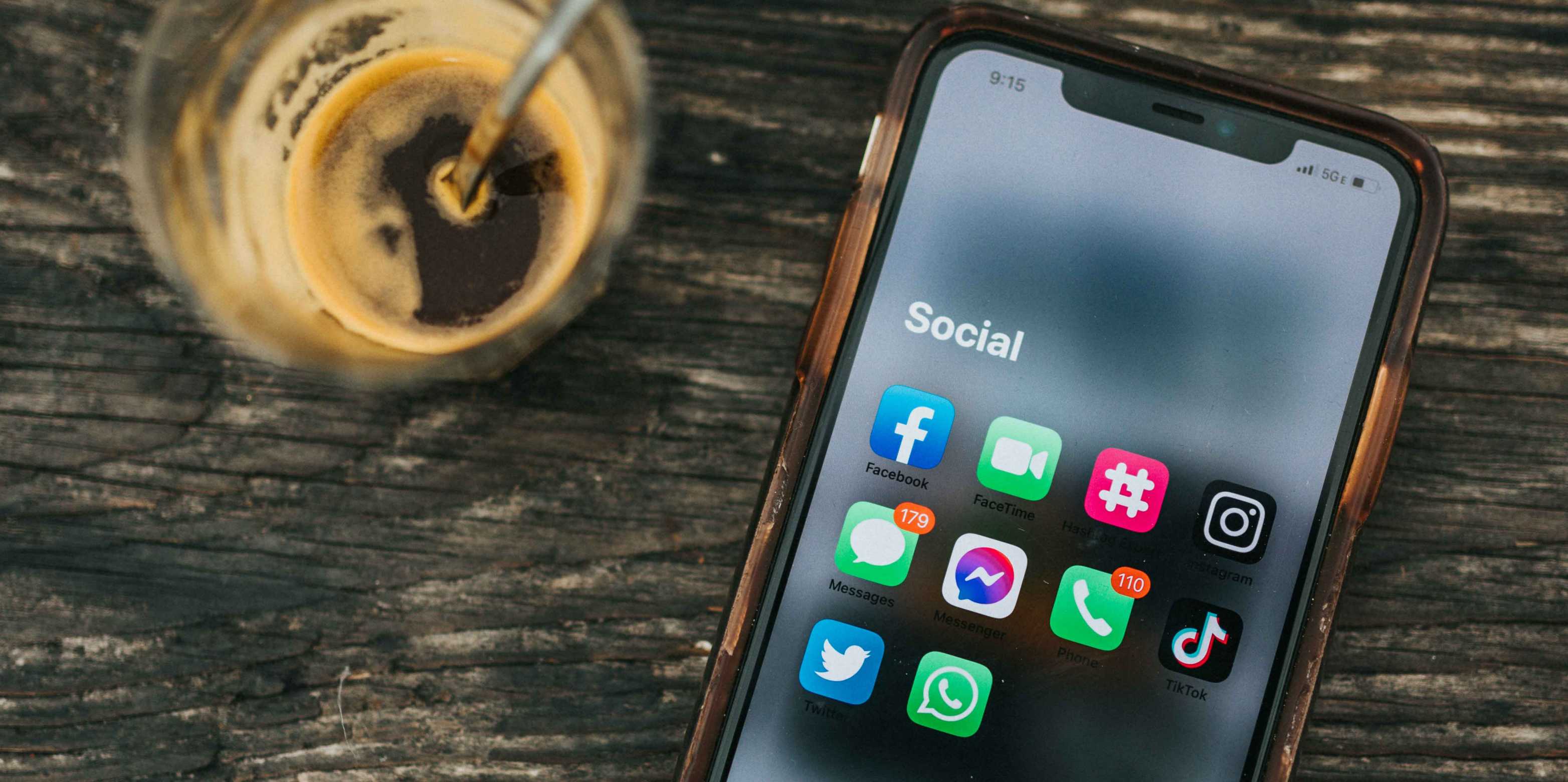
Understanding the Pulse of Singapore
Using social media data to analyse people's sentiments through Sophisticated AI Modelling.
Project Summary
- A collaboration between NTU, SEC, and MCCY in Singapore from November 2023 to May 2024.
- Study to understand whether sentiments can be gleaned from social media to support analysis on the level of cohesion and care.
- Employing advanced AI techniques like large language models for data augmentation and training classifiers.
The external page Ministry of Culture, Community, and Youth (MCCY) is a ministry in the Government of Singapore dedicated to building a resilient country, and a cohesive and caring society. To better understand Singaporeans’ sentiments and concerns around issues such as social cohesion, MCCY regularly conducts sentiment surveys.
Researchers, led by external page Assoc. Prof. Dr Kezhi Mao from the external page Nanyang Technological University (NTU Singapore), and the Singapore-ETH Centre (SEC), led by Dr Jonas Joerin, approached MCCY to study how online forum discussions might add to understanding of public sentiments. To help make sense of the large volume of discussions occurring in the online space, the researchers developed a process to sieve out topics of interest, resulting in a less time-consuming method for a quick sense of online discussions.
Social media platforms have become a way for the public to engage in discussion and express their opinions on various issues. Beyond traditional surveys, new AI techniques allow us to analyse data from these social media platforms and offer insights into public perceptions around key topics of interest.
The researchers faced two significant challenges while developing new AI techniques. The first challenge involved identifying valid or relevant posts and comments from the vast expanse of social media data. The second challenge arose when the trained models encountered new data over time, leading to a problem known as domain shift. Specifically, new comments from the public may exhibit different characteristics and distributions compared to the data initially used for training. This shift necessitated regular updates to classifiers to maintain their reliability and adaptability.
To address the first issue of relevant comment identification, researchers began by annotating a small seed dataset for each specific topic. They employed Large Language Models (LLMs) to create artificial data, thereby enhancing the dataset through data augmentation. This augmented data is used to train robust classifiers for each topic, which help in gathering and analysing public comments. Each topic is further segmented into fine-grained subjects; for instance, national cohesion is divided into discussions on religion, race, socioeconomic status, etc. A binary classifier (this topic/not this topic) is then trained for each of the fine-grained topics to analyse the social media stream.
To mitigate the second issue of domain shift, they use a semi-supervised framework to incorporate newly published data, thus evolving the classifiers and enhancing their domain adaptability. These models can then analyse the social media stream, providing insights into public perceptions of the identified topics.
The study demonstrated the potential to leverage AI models to glean insights from social media and support understanding of public sentiments around issues such as social cohesion.