Understanding traffic congestion patterns with deep learning
FRS researchers examine how existing research detects, predicts and alleviates traffic congestion
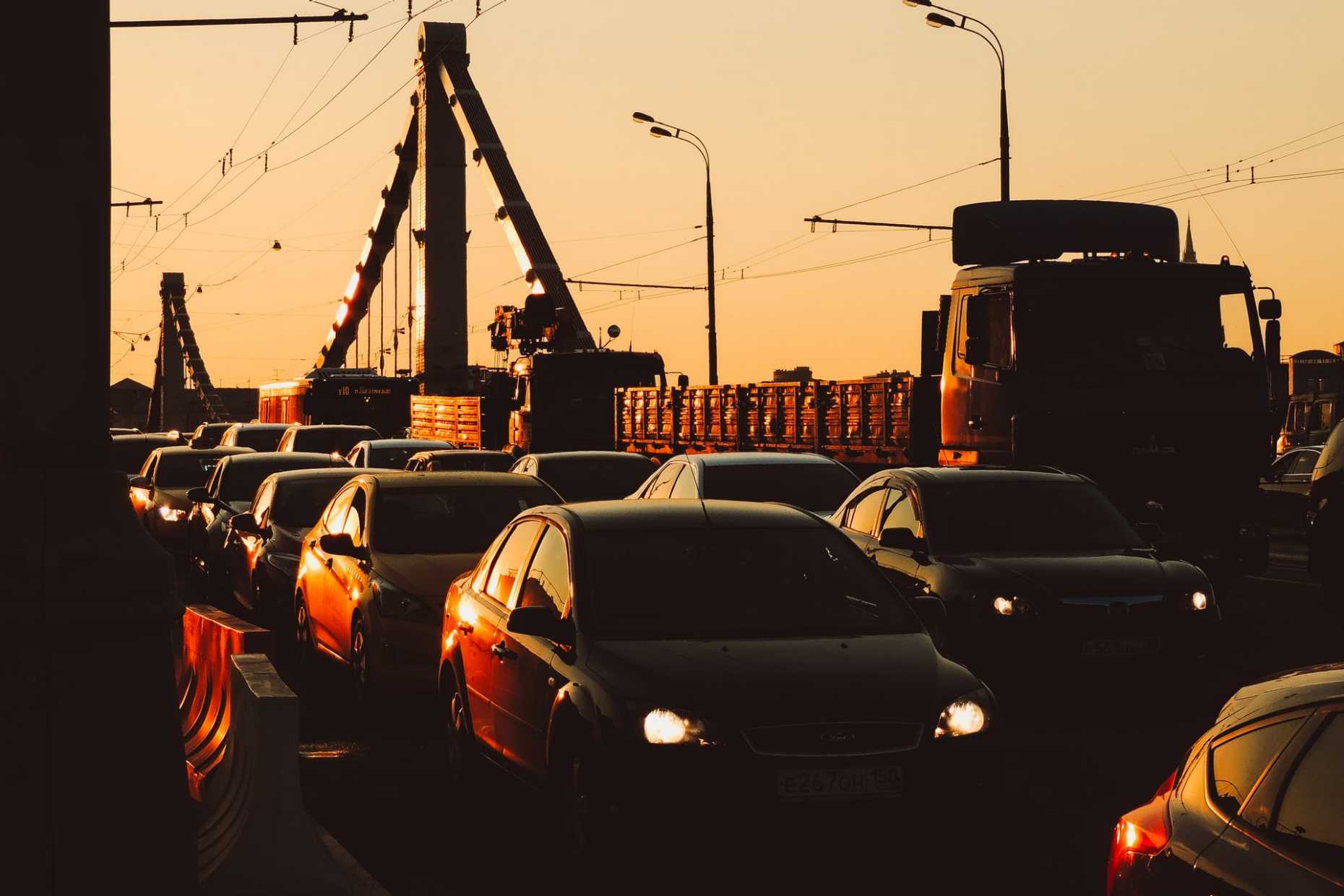
How can deep learning be applied to manage traffic congestion and to make road travel safer and faster at the same time? Can these objectives be achieved while ensuring fairness across individuals or groups? Are there potential conflicts of interest if a small number of private players cater to the route suggestion requirements for the majority of the population?
Researchers Nishant Kumar and Prof. Dr. Martin Raubal of FRS address these questions and more in their paper, 'Applications of deep learning in congestion detection, prediction and alleviation: A survey'. Examining the current state of deep learning applications in the tasks of congestion prediction, they also look at gaps and outline challenges for future researchers.
In their paper, they look at three specific areas:
- In the first part, they study congestion detection, summarising how the deep learning models that were initially developed for computer vision tasks are being applied in detecting traffic congestion. A clear distinction is seen between the models and data sources used in different geographical locations.
- The second part addresses congestion prediction. They explain why congestion prediction is a more difficult task than traffic prediction. They also discuss specific examples of how researchers have leveraged the domain knowledge from transportation, such as the heterogeneity of road networks, to improve the overall prediction accuracy of deep learning models.
- Finally, their third part investigates congestion alleviation. They highlight how deep learning is being used for alleviating congestion. The paper reviews the challenges in implementing demand-side solutions argue in favour of system-level optimizations with the policymaker in the loop.
The paper concludes by drawing attention to the potential clash of interests between efforts to alleviate recurrent and non-recurrent congestion. Their analysis is recorded as the hypothetical U-curve below showing the current understanding of how traffic congestion and road safety are related, based on their research survey.
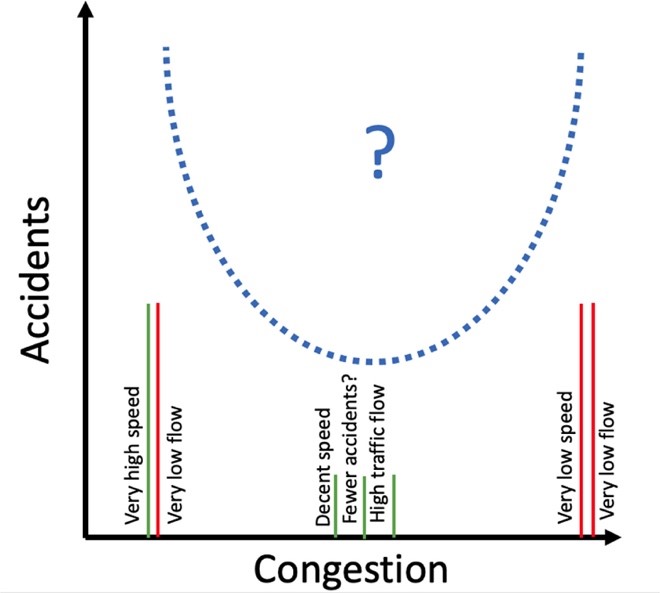
Nishant Kumar, Martin Raubal, Applications of deep learning in congestion detection, prediction and alleviation: A survey. Transportation Research Part C: Emerging Technologies, Volume 133, 2021, 103432. external page https://doi.org/10.1016/j.trc.2021.103432.